DIB-R++: Learning to Predict Lighting and Material with a Hybrid Differentiable Renderer
In Neural Information Processing Systems (NeurIPS), December 2021
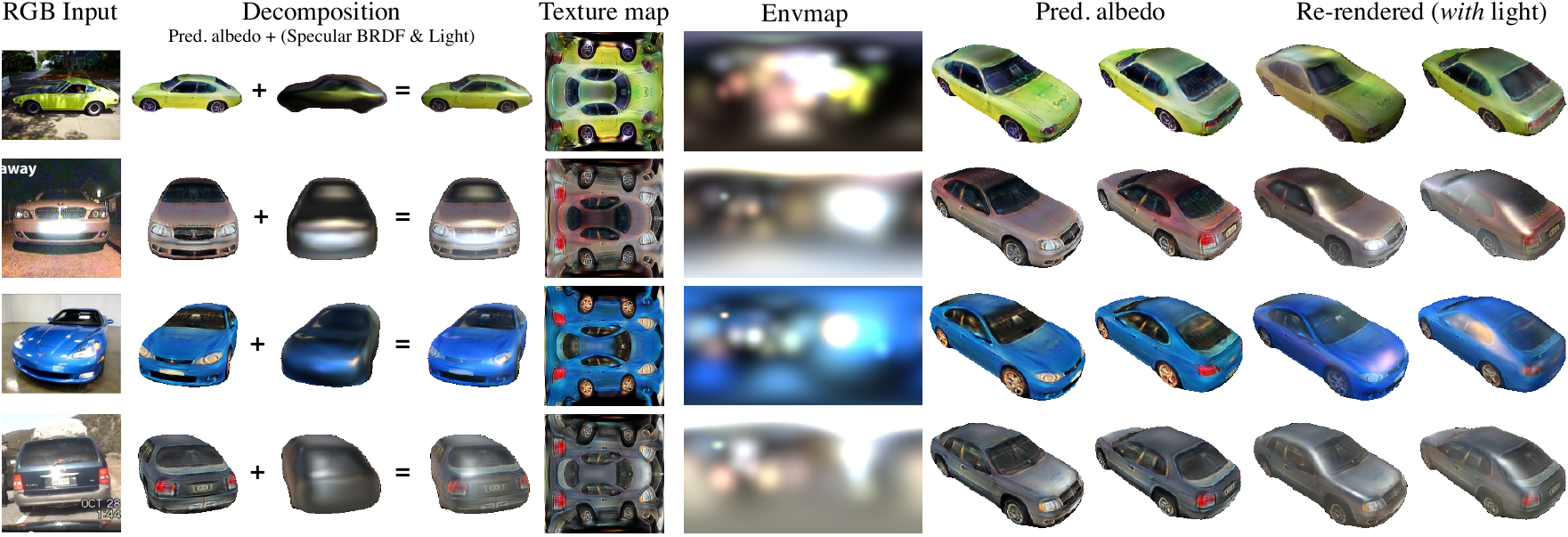
Prediction on LSUN Dataset (Cars): DIB-R++, trained on StyleGAN dataset, can generalize well to real images. Moreover, it also predicts correct high specular lighting directions and usable, clean textures.
Abstract
We consider the challenging problem of predicting intrinsic object properties from a single image by exploiting differentiable renderers. Many previous learning-based approaches for inverse graphics adopt rasterization-based renderers and assume naive lighting and material models, which often fail to account for non-Lambertian, specular reflections commonly observed in the wild. In this work, we propose DIB-R++, a hybrid differentiable renderer which supports these photorealistic effects by combining rasterization and ray-tracing, taking the advantage of their respective strengths—speed and realism. Our renderer incorporates environmental lighting and spatially-varying material models to efficiently approximate light transport, either through direct estimation or via spherical basis functions. Compared to more advanced physics-based differentiable renderers leveraging path tracing, DIB-R++ is highly performant due to its compact and expressive shading model, which enables easy integration with learning frameworks for geometry, reflectance and lighting prediction from a single image without requiring any ground-truth. We experimentally demonstrate that our approach achieves superior material and lighting disentanglement on synthetic and real data compared to existing rasterization-based approaches and showcase several artistic applications including material editing and relighting.
Downloads
Publication
- Paper (author's version), PDF (2.9MB)
- arXiv version – external link
- Publisher's official version – external link, may require a subscription
- Primary website – external link
BibTeX
Cite
Wenzheng Chen, Joey Litalien, Jun Gao, Zian Wang, Clément Fuji Tsang, Sameh Khamis, Or Litany, and Sanja Fidler. DIB-R++: Learning to Predict Lighting and Material with a Hybrid Differentiable Renderer. Neural Information Processing Systems, X (X), Article XX, December 2021.
@inproceedings{Chen:2021:DIBRPP, title = {{DIB-R++}: Learning to Predict Lighting and Material with a Hybrid Differentiable Renderer, author = {Wenzheng Chen and Joey Litalien and Jun Gao and Zian Wang and Clement Fuji Tsang and Sameh Khalis and Or Litany and Sanja Fidler}, year = {2021}, journal = {Conference on Neural Information Processing Systems (NeurIPS)} }
Copy to clipboard